Health & Fitness based mobile apps need large amounts of granular data from its users for better processing and accurate recommendations.
The ‘HOME’ solution by NimbleEdge enhances on-device data-processing capabilities that work at scale can improve user experience and business revenue.
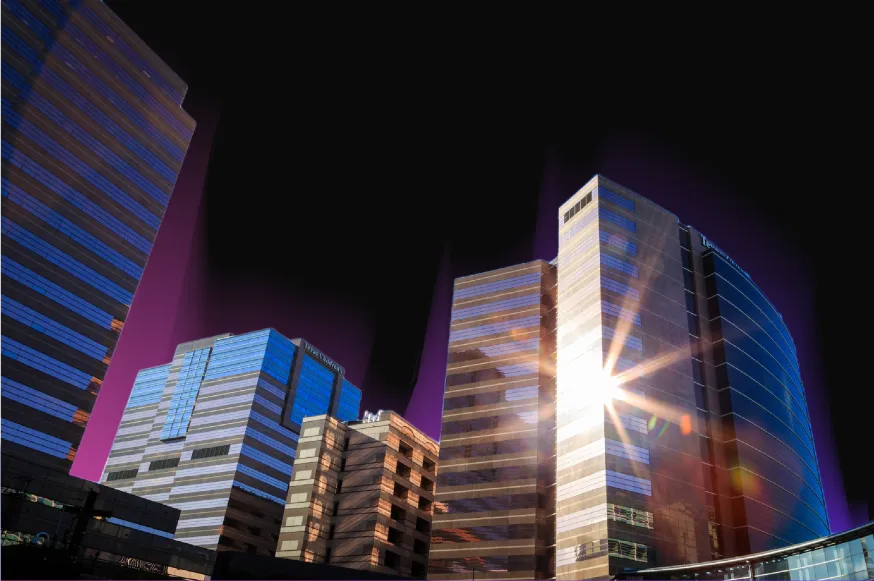

‘Health & Fitness’ based mobile apps that try to deliver accurate and personalized recommendations need large amounts of granular data from its users for better processing and accurate recommendations. Large data processing in health & fitness brings two problems as a subset - user’s sensitive personal identification information (PII) being risked and high processing costs. Thus, cloud systems by their very design, cannot support health-based mobile enterprises to deliver highly privacy-preserved solutions with lesser processing costs.
NimbleEdge comes into the picture to enable data processing at extremely granular levels while keeping user’s PII completely safe. Its enhanced on-device data-processing capabilities that work at scale can improve user experience and business revenue.
Personalized Search recommendations & Health-monitoring models

Real-time ML processing on the Cloud is impossible due to escalated data risk, limited scalability, and increased costs.
Personalization, privacy, and processing costs have been fighting against each other when it comes to ML processing for Healthcare related mobile apps. As a result, user engagement metrics, customer acquisition and retention, NPS, and other business app metrics suffer. On-device/Edge processing can be a great solution but the data processing capacity is inherently limited due to resource constraints of edge devices.

Bringing on-device real-time processing capabilities at 1/5th of the cloud costs.
NimbleEdge’s HOME runs real-time ML - Inference & Training - on-device, ensuring performance uplifts in Personalized Search recommendations and Health monitoring models at 1/5th of the cost to run them on the cloud. Imagine the right exercise, pose, and nutrition being recommended to the user after they have finished working out. Hence, real-time ML offers reduced latency and better results, cascading improvements in other business metrics of the app.
Real-time ML offers reduced latency and better results, cascading improvements in other business metrics of the app.
Privacy budget savings - ~70% savings in the privacy budget for major processing done on-device with privacy preserving encryption algorithms. User’s sensitive PII is completely safe.

~10-20% improvement in the model’s performances lead to
Uplifts in click-throughs, acquisition, retention, and lifetime value of the user.

~33-80% - massive cloud cost savings from the existing ML infra. Significant bottom-line savings.

NimbleEdge’s Platform provides both orchestration and execution capabilities for Hyper-Personalization.
on-the-fly updates
Orchestration
PaaS
Leverage the Intelligent Edge for Your Industry
Want to
learn more?
