ML processing on the cloud is no longer sufficient to navigate the challenges faced by FinTech mobile apps
NimbleEdge’s HOME runs on-device Inference & Training for large fraud & personalization models, thus leading to great accuracy uplifts at 1/5th of the cost in running these models.
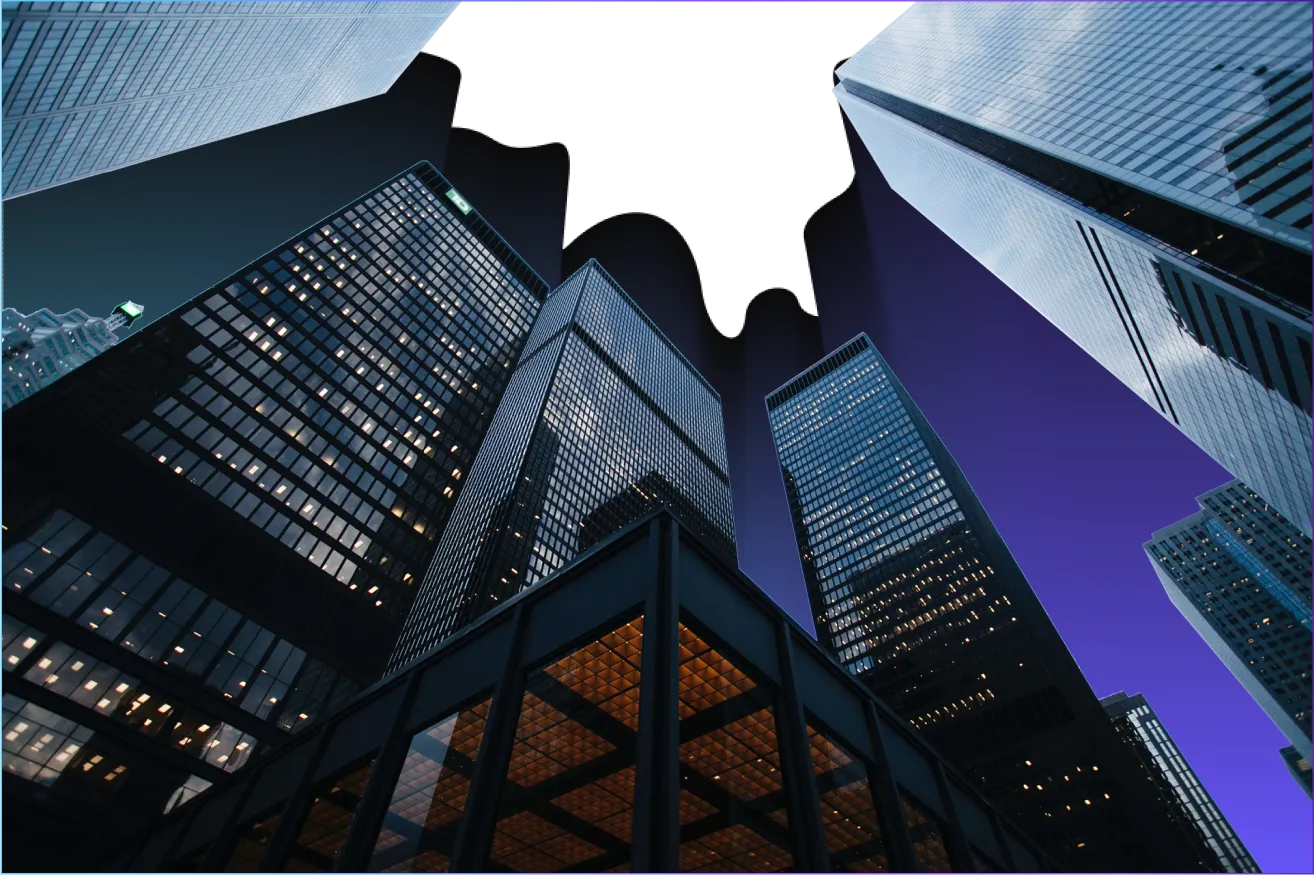
ML processing on the Cloud is no longer a solution for FinTech mobile apps managing payment transfers, payment gateways, lending personal loans, investments and insurance.
The cloud ecosystem limits the potential of FinTech apps in providing a fast, reliable, and Hyper-Personalized user experience.
NimbleEdge comes into the picture to help you take your speed, reliability and user personalization metrics to new benchmarks with their Mobile Edge based solution called ‘HOME’. It has the capabilities to do on-device ML processing at a much bigger scale than cloud systems could imagine!
Fraud detection models that try to flag fraudulent transactions
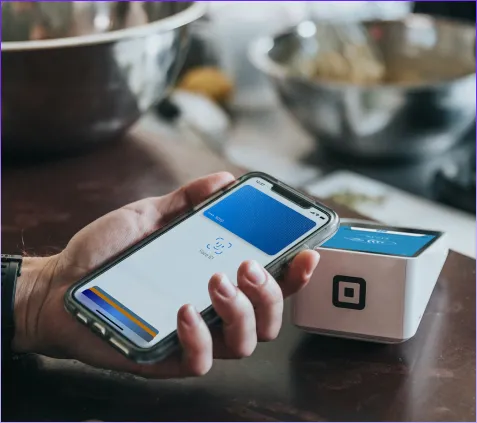
Speed & Reliability issues with transactions in non-real time ML systems on the cloud limit personalization levels as it operates with stale data i.e. lagging data for AI/MLHuge Costs of running real-time ML systems on the Cloud
worth of transactions are unsuccessful due to fraud and false decline flaggings, in the USt.
High response times of a minimum of 500ms-1500ms for a complete transaction 77% of the customers believe that real-time individualization/personalization is key for a great customer experience
Be it real-time or non-real-time ML Cloud-systems, sending customer data to a far-located server creates risk with fraud, speed, and reliable completion of transactions. With respect to recommendations of services/products/rewards, they don't always get the user’s personal interests accurately due to limits in effectiveness of personalization algorithms as the data is not granular but a product of 12-24 hours old batch processing. Granular data can be leveraged with on-device (user’s mobile devices) model deployment and processing but it is not practically possible due to the operational complexities of Edge processing - scalability & resource handling of the mobile devices.

Bringing models and ML data processing to the mobile devices at an infinite scale
NimbleEdge’s HOME runs on-device Inference & Training for large fraud & personalization models, thus leading to great accuracy uplifts at 1/5th of the cost in running these models. It also runs multiple models on the Edge for even a billion transactions at a time. Hence, providing reliable, fast and hyper-personalized transaction experiences at significantly lower costs!
Complete travel bookings without leaving the app, saving huge amounts of retargeting advertising expenses
~10-20% improvement in the model’s performances lead to
Betterment in transaction success rate through hyper-personalized fraud detection

~10-20% improvement in the model’s performances lead to
Highly individualized product/service recommendations, leading to high customer retention and NPS metrics

Huge Topline growth due to more successful transaction numbers!

<20ms - minimum 25x faster transaction response time that lead to uplifts in NPS

~33-80% - massive cloud cost savings from the ML infrastructure

NimbleEdge’s Platform provides both orchestration and execution capabilities for Hyper-Personalization.
on-the-fly updates
Orchestration
PaaS
Leverage the Intelligent Edge for Your Industry
Want to
learn more?
